Data Quality in Healthcare: Importance & Examples
Healthcare organizations are increasingly using data to improve decision-making. However, to perform accurate data analysis, it is crucial to ensure the data being used is of sufficient quality. Poor healthcare data quality can lead to not only a loss of revenue but also put patients’ lives at risk.
Oftentimes just a single erroneous piece of data in a provider’s EHR system can lead to improper treatment and can negatively affect patient outcomes. Considering that patient data is highly sensitive information and requires strict regulatory and security measures, there’s a need for well-established healthcare data quality management in the industry. By complying with regulations such as HIPAA and establishing data quality protocols, providers can protect sensitive patient information while leveraging the benefits of data analytics for healthcare.
In this article, we’ll explore the importance of data quality in healthcare organizations, discuss how data standards facilitate data quality, and talk about how to prevent healthcare data quality issues.
The Importance of Data Quality in Healthcare
Nowadays, data quality management in healthcare is crucial for all organizations that work with data, regardless of whether their operations involve sharing, analyzing, or managing data. However, when it comes to healthcare providers who deal with patients’ medical records that contain sensitive information daily, the importance of data quality becomes even more significant. The sensitive nature of healthcare data demands that regulations and strict security measures are put in place to ensure that patients’ privacy and confidentiality are protected.
To truly grasp why data quality is important in healthcare, providers must first understand the implications of accessing medical data regardless of its use case. Healthcare providers must understand that obtaining a patient’s medical information, including reports and records, can significantly affect both the individual and the treatment they are provided.
For example, the accuracy and completeness of the medical data can directly impact the accuracy of the diagnosis and the effectiveness of the treatment. Recurring errors resulting in injuries or even death are often caused by poor data management practices, primarily due to mistakes in recording or transcribing.
As such, the role of data quality in healthcare information systems is key to improving patient outcomes. By ensuring that healthcare data is accurate and complete, providers can make better-informed decisions, offer improved treatment options, and lower the likelihood of medical errors. It’s important to have quality data in healthcare, as it ultimately leads to increased patient satisfaction, better care, and improved patient outcomes.
How Is Healthcare Data Quality Measured?

A poorly maintained data quality can result in more bottlenecks, lower efficiency, and potential patient mistreatment. This is where an effective data quality management system comes into play, helping improve data accuracy and reliability. Such systems are based on the following data quality metrics:
- Accuracy.
High-quality data should be correct and free from errors or inconsistencies. Data precision and accuracy depend on how the data will be used; thus, it’s important to understand the purpose of data to avoid wasted resources and off-target information.
- Validity.
Data validity pertains to specific requirements set for data types, like surveys with predefined answer options for ethnicity, gender, or nationality. Incomplete or incorrect answers are considered invalid, which is crucial in determining data quality. Hospital departments have their own data rules to distinguish relevant data from irrelevant ones.
- Reliability.
Data collected by multiple systems may have originated from different sources, but a steady mechanism must ensure consistency across data collection and storage. This is to avoid unwarranted variances that could affect data quality, regardless of the data source or storage location.
- Cohesiveness.
Inaccurate and incomplete data are equally problematic, with missing information creating a distorted view that does not reflect reality. Without a complete understanding of operations, uninformed decisions are likely to occur, leading to inefficiencies in the organization.
- Uniqueness.
Data uniqueness refers to the level of duplication within or across systems for a specific field, record, or data set. A higher uniqueness score indicates fewer duplicates or overlaps, increasing confidence in data and analysis. Recognizing overlaps can aid in preserving the uniqueness and removing duplicate records through data cleansing, and deduplication can help mitigate these issues.
- Timeliness.
In healthcare, timely and accurate data is crucial for providing patients with the best possible care. Delayed or outdated information can lead to incorrect diagnoses, inappropriate treatments, and even patient harm.
HL7 Standards and Data Quality. What Do They Have in Common?
Healthcare data standards are an essential part of healthcare data management; they help ensure patient data is stored and exchanged accurately. Some of the most widely used healthcare data standards are those developed by HL7 International, a non-profit accredited by ANSI and dedicated to developing standards aimed at promoting the interoperability of health data on a global scale. These standards include FHIR (Fast Healthcare Interoperability Resources), CDA (Clinical Document Architecture), and HL7 v2 standards. The main goal of data standardization is to establish a reliable way for how data is stored and exchanged between systems by setting specific regulations on how data is transmitted.
HL7 standards facilitate data and documentation consistency across all systems, regardless of the organization they are part of. This means that medical professionals can easily access patient data, no matter what health information system they are employing, thus making it easier and more efficient to provide higher-quality care.
Additionally, an FHIR server can help with data validation by providing tools and functionality to ensure the data being exchanged between healthcare systems and applications meets the requirements defined by the standard. This includes validation of data types, structure, and code systems.
An FHIR server also validates data against profiles (a set of rules about how the elements in an FHIR resource are used and where extensions are used in an FHIR resource.) In that case, an FHIR server will check the following:
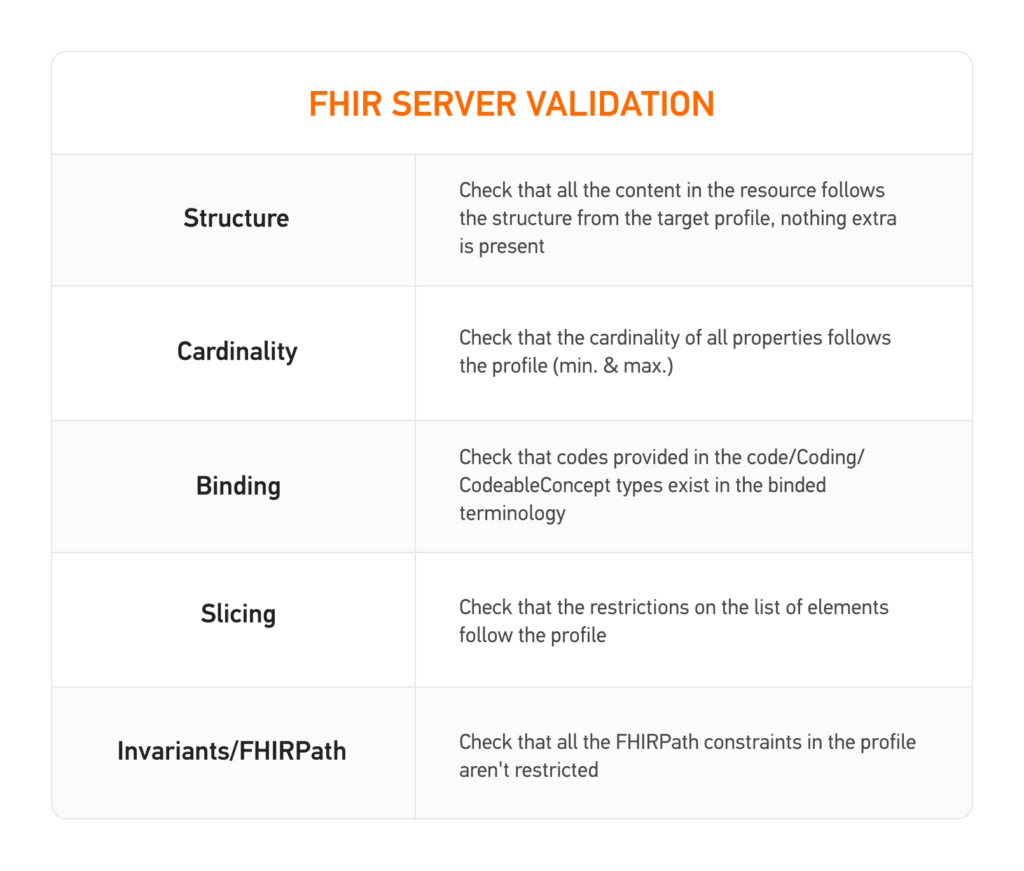
Thus, the data received by an FHIR server will be accurate and complete. By providing robust data validation capabilities, FHIR servers help improve the quality of healthcare data and promote interoperability between different healthcare systems and applications.
HL7 standards integration helps healthcare providers ensure their data is accurately recorded, exchanged, and stored, making it easier to apply data analytics for improved patient outcomes.
Examples of poor data quality in healthcare
Here are some examples of data quality issues in healthcare:
1. Inaccurate or Incomplete Patient Information: Data entry errors or incomplete documentation can lead to incorrect patient information, such as incorrect names, addresses, or medical history. This can have serious implications for patient care and treatment.
2. Duplicate Records: Duplicate records occur when multiple entries are created for the same patient, often due to inconsistencies in data collection or inadequate data matching algorithms. This can result in fragmented and incomplete patient information, leading to potential errors in diagnosis, treatment, and medication administration.
3. Inconsistencies in Terminology and Coding: In healthcare, different terminologies and coding systems are used across various departments and systems. Inconsistent use or interpretation of these terms can lead to confusion and errors in data analysis, research, and decision-making.
4. Data Integration Challenges: Healthcare organizations often collect data from various sources, including electronic health records, medical devices, and administrative systems. Integrating these disparate data sources can be complex and challenging, leading to data quality issues such as data inconsistency, duplication, or missing data.
How to Overcome Data Quality Issues in Healthcare
Improving data quality in healthcare is critical to ensuring accurate and reliable data for decision-making processes. Below we will examine how to address these examples of data quality issues in healthcare.
Through various strategies, healthcare organizations can improve the reliability and usefulness of their data, leading to better patient care and informed decision-making.
Data Governance
Establishing data governance policies and procedures ensures data is managed in a consistent and standardized way across the organization. This includes creating policies on data collection, data storage, data sharing, and data quality control.
Data Standardization
Standardizing data elements such as terminology, coding, and formatting can help ensure data is consistent and accurate across different systems and departments.
This is where FHIR can help. As mentioned previously, it provides a set of resources and data elements that are standardized and widely adopted, allowing for consistent representation of healthcare data across different systems and organizations. These resources cover various aspects of healthcare, including patient demographics, clinical observations, medications, procedures, and more.
By leveraging FHIR, healthcare organizations can achieve data standardization by following the defined data models and structures provided by the FHIR standard.
Data Cleaning
Regularly cleaning data by removing duplicates, correcting errors, and filling in missing data can help improve data quality.
Data Validation
Validating data as it is entered into the system can help reduce data entry errors and ensure data is accurate and complete.
Kodjin FHIR Server offers features that enable data validation during data entry, ensuring that the entered data adheres to the defined standards and rules.
When data is entered into the Kodjin FHIR Server, it can perform validation checks against the FHIR specification and the specific profiles and constraints defined for the resources. This validation process verifies the integrity and quality of the data being entered.
Staff Training
Providing staff with training on data collection, data entry, and data quality control can help improve data quality and ensure data is collected and managed consistently across the organization.
Technology
Implementing technology solutions, such as electronic health records (EHRs) and clinical decision support systems, can help improve data quality by automating data collection and reducing data entry errors.
Data Audits
Conducting regular audits of data and continuous data quality monitoring can help identify and correct data quality issues before they become a problem.
Data quality tools in healthcare that are used
Data quality improving tools play a vital role in healthcare organizations by improving the accuracy, completeness, and consistency of healthcare data. These tools help identify and address data quality issues, ensuring reliable and trustworthy information for decision-making processes.
Quality tools in healthcare encompass a variety of functionalities that contribute to data quality improvement. These tools often integrate with information systems, including electronic health records (EHRs) and other healthcare databases, to facilitate data validation, cleansing, and standardization.
One such tool is the Kodjin FHIR Server, which offers a range of data quality improving features. By utilizing Kodjin FHIR server, healthcare organizations can simplify their data management processes and ensure the accuracy and reliability of their healthcare data.
Kodjin serves as a secure and scalable platform for storing and managing various types of healthcare data, including patient records, laboratory test results, and medical images. It provides a standardized approach to data storage and exchange, making it easier for healthcare systems to integrate and share information.
When data is transformed into the FHIR format and stored on the Kodjin FHIR server, it undergoes a validation process against FHIR profiles. These profiles define the structure and constraints that the data should adhere to. By validating the data against these profiles, the server helps identify any inconsistencies or errors, ensuring that the data is compliant with the FHIR standard.
Furthermore, the Kodjin FHIR server allows for the updating of profiles. Meaning that when a new profile is created or an old profile is updated, Kodjin will validate the resource using new validation rules. This enables continuous validation of data and ensures ongoing accuracy. Schedule a free demo with us to learn more about how Kodjin can help you maintain and improve data quality within your organization.
Conclusion
Data quality is essential for healthcare organizations to make informed decisions and provide patients with the best possible care. Accurate and timely data can help healthcare professionals identify patterns, predict outcomes, and improve patient outcomes. Poor data quality in healthcare, on the other hand, can lead to incorrect diagnoses, inappropriate treatments, and patient harm.
To ensure data quality in healthcare, organizations need to invest in data governance, data management, and data analysis tools and continuously monitor and improve data quality.
If you’re working with FHIR-compliant data or want to adopt the FHIR standard, it’s important to pick a smart FHIR server that can automatically validate your data to meet the specification’s requirements. The Kodjin FHIR Server provides dynamic profiling with advanced validation capabilities, including structure, cardinality, binding, slicing, and FHIRPath. Schedule a free demo with us to learn more about how Kodjin can help you maintain and improve data quality within your organization.
Conclusion: So above is the Data Quality in Healthcare: Importance & Examples article. Hopefully with this article you can help you in life, always follow and read our good articles on the website: W Tài Liệu